Who’s really behind the wheel?
WBJ talked to Paweł Rzeszuciński, PhD, Chief Data Scientist at Codewise about AI ethics in autonomous cars
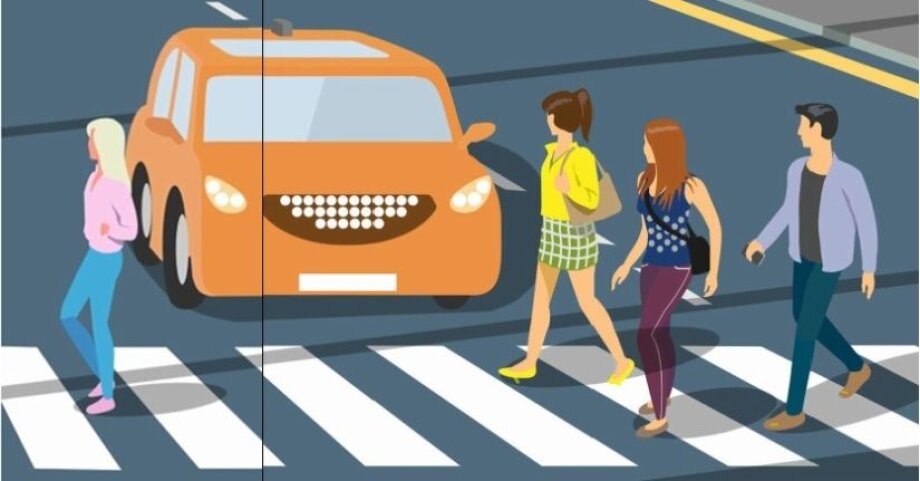
WBJ talked to Paweł Rzeszuciński, PhD, Chief Data Scientist at Codewise about AI ethics in autonomous cars
Should an autonomous car avoid hitting a pedestrian at all costs? If an accident cannot be avoided, who should it choose to save: a child or a group of jaywalkers? What about control – when should a human driver be given control of a vehicle? And how are autonomous vehicles protected from hackers? These are some very important questions that the automotive industry has yet to find answers to.
Interview by Beata Socha
WBJ: Currently, in the case of an emergency, autonomous cars release control of the vehicle to the driver (or simply brake). This has obvious drawbacks, as the response time of an idle driver will definitely be longer than that of an active one. Do autonomous car producers have any plans to solve this problem?
Paweł Rzeszuciński: It is correct that in the advanced driving assistance systems that are currently available the default behavior is to either brake or request that the driver take over control in an emergency situation; however, the entire philosophy of fully autonomous vehicles (AVs) is to be able to act in a way that is the most appropriate in a given situation (which sometimes may be neither of the two mentioned above).
In order to get a glimpse of how advanced the recent developments are, I’d advise searching on YouTube for compilation videos of how autonomous car autopilot systems predict dangerous situations on the road and the variety of ways they react (bear in mind that an autopilot is just a semi-autonomous system).
Today’s AVs are equipped with an abundance of different sensor types designed to accurately describe the situation around the vehicle. These include cameras, radars, lidars and ultrasonic sensors. These provide the “senses” of the AVs and give them the opportunity to understand the situation on the road and assess the best possible course of action to avoid accidents.
In addition to what’s already available, there are options for further improving the safety capabilities and inter-AV communication. These include Vehicle-to-Vehicle communication systems (V2V), wireless networks where AVs communicate with each other, exchanging updates (speed, location, direction of travel, braking and loss of stability); Vehicle-to-Infrastructure (V2I) in which elements of the infrastructure feed AVs with real-time data about road conditions, traffic congestion, accidents or construction zones; Vehicle-to-Environment (V2E) which can, for example, inform AVs about the positioning of pedestrians or cyclists.
With the introduction of these new communication networks, the vehicles will become even better informed about the current situation on the road and will be given much more insight into how to best respond to predicted hazards.
In addition to sensor-based reasoning, the industry is working on providing some “common sense” instructions aimed at increasing the safety of AVs. A good example might be Mobileye, which Intel acquired for $15.3 billion in 2017, a company that came up with Responsibility-Sensitive Safety (RSS) approach to on-the-road decision-making that codifies good habits. As stated by Intel, the RSS model does not allow an AV to mitigate one accident with another presumably less severe one. In other words, in attempting to escape a collision caused by a human driver, the RSS model allows the AV to take any action (including violating traffic laws) if those actions do not cause a separate accident. This constraint is appropriate because the judgment of accident severity is subjective and might miss hidden, critical variables, such as a baby in the back seat of the seemingly “less severe” accident.
It seems that saving lives should be the ultimate aim in the case of collisions. Is there a universal code of ethics that could govern AIs in such cases?
A paper published at the end of last year in Nature shed some light on the results of the Moral Machine quiz – an online questionnaire that presented participants with a set of nine ethical choices regarding fictional driving scenarios. The quiz – in the form of the famous trolley problem – was taken by more than two million people from 233 countries. The questions the respondents had to answer included choosing to kill pedestrians or jaywalkers, young people or the elderly, and women or men.
Even though the trolley problem in itself is not the best representative of the challenges of the AV industry, it is a useful example as to how people may react to the advent of new technologies. As the report outlines, in general the respondents would save more lives over fewer, children over adults, and humans instead of animals. However, the more granular answers differed across different geographical, ethnic, and socio-economical regions.
For example, participants from countries with low GDP were much less likely to sacrifice a jaywalker compared to respondents from countries with stronger economies. At the same time, residents of Asia and the Middle East were more reluctant to spare young people over older pedestrians and were more likely to protect wealthier people compared to quiz-takers from North America and Europe.
These results show that it would be pretty much impossible to devise ethics rules that would be universally acceptable worldwide. Luckily for us, however, the decision-making process of modern AI systems is not a black-white type of choice, but rather a result of complex optimization, always looking for the best solution given the circumstances.
It is only natural that a driver faced with an impending collision will try to avoid it and protect his or her own life. What would an AI do in this case?
When analyzing the Google search trend for phrase “autonomous cars ethics” there are virtually no instances from before the end of 2013. Six years to solve such a complex task seems like a very short period of time. Luckily, an increasing number of scientific bodies and companies are working on trying to find the one-size-fits-all solution. In essence, there are two main approaches. The more philosophical ones are based around trying to address the many aspects of the trolley problem. Luckily not all of the endeavors are based purely on variations of thought experiments.
Nicholas Evans, a philosopher from University of Massachusetts Lowell, gathered a group of fellow philosophers and engineers and received a $500,000 grant to write algorithms based on various ethical theories to test which are best suited for the trolley problem.
The research team is also interested in some follow-up questions e.g. how, under a given theory, a self-driving car could be hacked. For example, if a car was known to avoid pedestrians even at the cost of putting the driver at risk, someone could potentially put themselves in the path of the autonomous vehicle to harm the driver. More extreme cases, not unheard of by airplane pilots, could involve wrong-doers using infrared lasers to try to confuse the car’s sensory system.
Engineers, on the other hand, often look at the trolley problem as an idealized, unsolvable decision-making problem which is often misleading to the entire process of how modern AI systems make decisions. Due to the way the problem is stated, it has a limited number of possible answers, all ethically questionable and perceived as bad or wrong. In contrast, an engineering problem is always constructed in a way that a better and worse solution has to be clearly reachable. At the end of the day it will be up to the software to make the decision on how to act in each given scenario.
In the past, decisions that were to be made by computers were hard-coded in so-called recommender systems where, in simple terms, a collective knowledge of domain experts was hard-coded in a set of rules such as “if situation A happens, perform action B.” However, with the increasing complexity of systems, such an approach was no longer feasible. Instead, machines started to make judgements on their own by trying to reach an optimal solution based on a number of inputs, often of very different types. So, framing the optimization question in terms of simply “who to sacrifice” is a very big simplification of reality. AI systems will instead work towards causing the least damage by taking into account a very broad amount of input data, not only related to the vehicle itself, like its speed and direction, but also all the obstacles around it (humans, animals, buildings) as well as its location and context (maps, street signs) and the presence of other vehicles.
This all boils down to a very complex, multi-dimensional optimization task geared towards looking for the optimal solution under considerations of costs, quality and all the risk factors. Naturally, the question may arise as to how to define the “damage” to be minimized. Should the value of human lives be simply a single variable (e.g. derived from the Value of Statistical Life formula) and be considered as equally important as the value of cultural and environmental assets (the cost of destroying ancient heritage or polluting an important water reservoir)? There is no single best answer yet on the market, and in my opinion we are still a long way away from a universal standard that would be acceptable to all stakeholders.
There is still the question of responsibility. Who is responsible for a car accident caused by an autonomous car: the driver or car producers/AI designers?
With the amount of data that autonomous vehicles collect it will be an easy task to determine whether at the time of the accident, the human driver or the algorithm was making the decisions. Should the human driver be in charge, the classical liability as we know it today would be enforced. It becomes more interesting in cases where it was the algorithm that was driving the vehicle. In order to be able to determine whether the vehicle acted correctly, a unified set of rules should be defined that would allow us to assess whether the behavior was right or wrong. For this reason, some researchers suggest an introduction of a sort of “driving license” for autonomous vehicles that would test the reactions of the algorithms to some common situations, exactly as a human driver is during a driving license test.
Naturally, given the amount of possible circumstances on the road, it’d be impractical to conduct such tests entirely in a real environment, and so the great majority of the checking would have to be based on the software reactions to computer simulations. Should the algorithm of the autonomous vehicle fail in a given scenario, the testing body should demand an explanation as to what exactly failed and how a given decision was made.
Given the increasing complexity of the algorithms, providing such an explanation could be tricky at times. Many industries, for example the banking sector, do not allow the use of scoring algorithms in cases where each step of the decision-making process cannot be explained in detail. On one hand, it prevents banks from using more sophisticated approaches, but on the other hand it protects the interest of the clients. There are some that are calling for similar transparency requirements in the automobile industry but given that the only algorithms that are capable of dealing with the complex nature of driving a car are the least explainable ones (even for their creators) it might be a tough case to solve.
Can autonomous cars be safer than regular ones?
I see many analogies to the aviation industry and how its safety functions developed over time. The situation is akin to that of air crash investigations. I personally love watching the TV series “Air Crash Investigations,” which explains aviation-related disasters and crises, because virtually each episode is concluded with a list of improvements that have been made to improve the safety of the overall industry. When one analyzes the causes of crashes that took place in the distant past and the more recent ones, it becomes apparent how far the entire industry has moved to becoming the safest means of transportation on earth.
A very good practice has been introduced in California where all autonomous vehicles being tested must keep a continuous record of data from all their sensors for the 30 seconds prior to any incident or collision. This is virtually identical to the flight recorders (black boxes) on airplanes. This allows for every potentially dangerous situation to be reconstructed, analyzed and, if necessary, used to improve the behavior of the system. In essence, the amount of available data will make it possible to hold the car manufacturers accountable according to such higher safety standards that can be applied to humans.
It’s also worth noting that, most likely, autonomous vehicles will not be joining the traffic as we know it from the get-go. And imagining the introduction of AVs to our world as a sudden process seems far from realistic. Most likely, at the very initial stages, the deployment of AVs on a larger scale will be made possible only on dedicated lanes and in less crowded areas, to avoid difficult interaction with human drivers. Such a gentle introduction would give the manufacturers invaluable data to improve their systems, and it would allow humans to get used to the onset of the new types of road traffic participants.
What is the trolley problem?
It’s an ethical thought experiment where a participant has to choose between two bad outcomes of a traffic collision. Imagine you are driving a trolley and there is a group of five people on the tracks ahead of you. You cannot warn them, but you can switch the trolley to another track. However, there is also a person on the other track. There are several variations of the experiment, including one where a surgeon has five patients who will die without a transplant and a healthy traveler comes in for a check-up. The doctor can use his organs and save all five, but it would kill the traveler. The goal of the exercise is to determine a set of rules for what is ethically acceptable to people in a given society.
(wbj.pl)